Data Virtualization: A Smart Solution for Modern Hospitals
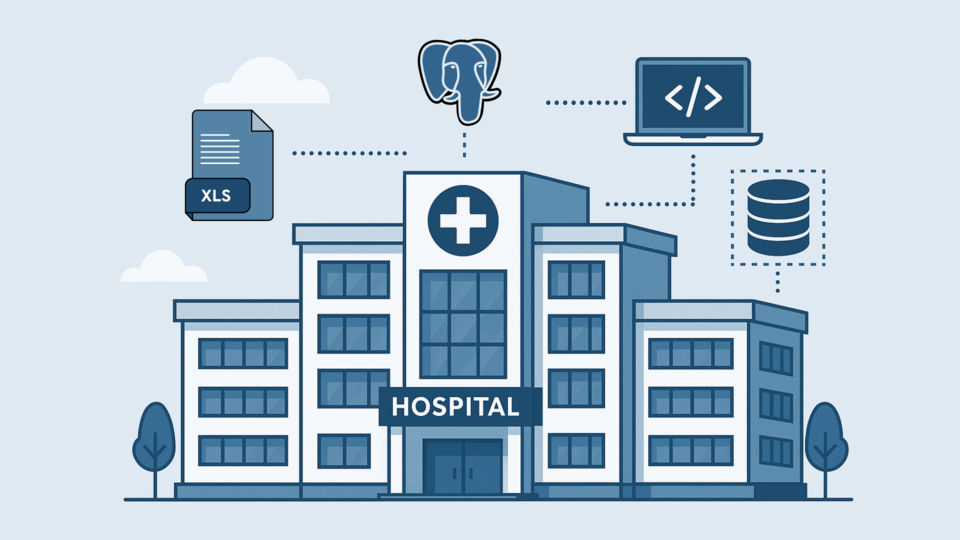
Every day, hospitals generate and store massive amounts of data from patient records and lab results to doctor schedules and electronic health records (EHR). Over time, legacy systems often become overwhelmed: slow, inefficient, and hard to access. That’s where data virtualization comes in as a modern solution. What is Data Virtualization? Data virtualization is a technology that allows hospitals to access multiple data sources through a unified view—without physically moving or copying the data. This means information can be accessed quickly and securely, even if it comes from different systems. Why Do Hospitals Need Data Virtualization? ✅ Faster & More EfficientDoctors and staff can access data from multiple systems in one place—no need to open various apps. ✅ Lower Risk of Data LossSince there’s no physical data transfer, the risk of data loss is significantly reduced. ✅ Reduced Infrastructure CostsNo need for massive servers or large IT teams. Virtualization saves space, time, and budget. ✅ Regulation & Audit ReadyWith cleaner, real-time data access, hospitals can more easily comply with healthcare data regulations and audits. ✅ Ideal for Legacy SystemsNo need to replace existing systems. Virtualization can work across both old and new systems simultaneously. Read Also : Data Migration in Healthcare: Challenges and Best Practices Data Virtualization vs. Data Migration Unlike data migration, which requires moving data into a new system, data virtualization connects to various data sources and displays them in real-time—no physical transfer needed. Feature Data Migration Data Virtualization Requires physical data move ✅ Yes ❌ No Real-time data access ⚠️ Limited ✅ Yes Works with legacy systems ❌ Not ideal ✅ Perfect fit Implementation cost 💸 High 💸 Cost-effective Operational risk ⚠️ High ✅ Low Deployment speed ❌ Slow ✅ Fast IT team required ✅ Large team ✅ Minimal effort Example: A Solution from Dave Platforms like Dave offer zero-ETL data virtualization, giving you access to all essential data without building complex pipelines or burdening your IT team. It’s a perfect fit for hospitals looking to digitize—without the hassle. Conclusion Data virtualization is a smart step forward for hospitals aiming to modernize without major disruptions. It’s faster, more cost-efficient, secure, and aligned with the demands of the digital healthcare era. 🔗 Explore the best data virtualization solutions for your hospital at https://hidave.io
Understanding Data Virtualization and Supported Sources
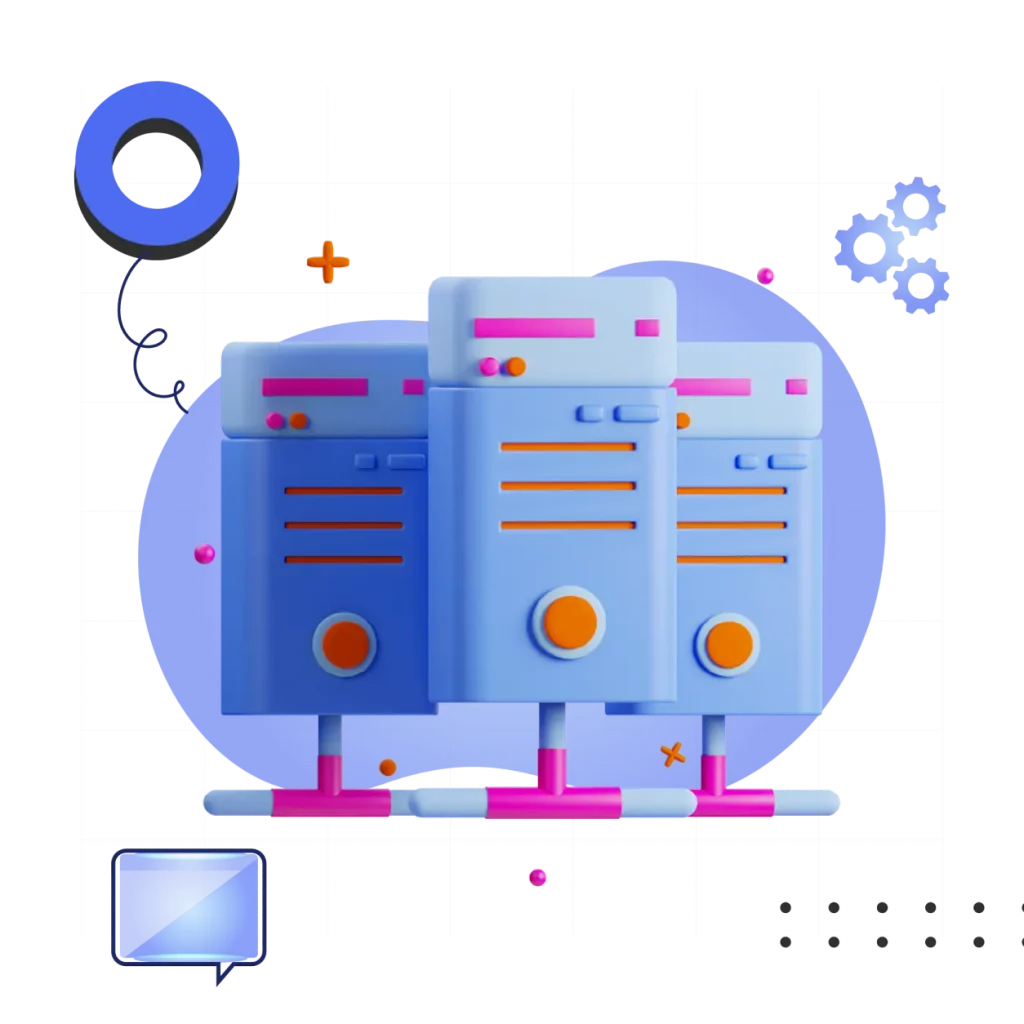
In the digital era, data has become a valuable asset for companies, but it is often scattered across various systems, formats, and platforms, making it difficult to manage. Virtualization offers a solution by enabling access and integration of data from multiple sources without the need for relocation or duplication. This technology creates an access layer that simplifies data management, allowing real-time analysis without altering the original sources. Use Case: Challenges Faced by Data Analysts in Data Integration A Data Analyst at a retail company is tasked with analyzing sales trends. The data needed comes from various sources: Before using virtualization, the team had to manually download and merge this data, which was time-consuming and prone to errors. With virtualization, the Data Analyst can access all this data in a unified view without relocating it, enabling faster and more efficient real-time analysis. Read Also : Supported data sources in Data Virtualization Supported Data Sources in Virtualization One of the key advantages of virtualization is its ability to connect with various data sources. Here are some of the data types that this technology can support: Diverse Data Sources Virtualization can connect various types of databases, both relational and non-relational, including: Various File Formats Besides databases, virtualization can also access and integrate data from different file formats, such as: Big Data Technologies In the world of big data, virtualization can connect with large-scale data processing technologies, such as: Benefits of Virtualization Faster Data Access: No need for physical data movement, which speeds up the analysis process. Cost Efficiency: Businesses can reduce the need to store data in separate systems, leading to significant savings. High Flexibility: This technology adapts well to various IT environments, whether on-premise or cloud-based. Enhanced Security: Since data remains in its original source, the risk of data breaches is minimized. Conclusion Virtualization is an effective solution for managing data from multiple sources without physically relocating it. With support for relational databases, NoSQL, file formats such as CSV and JSON, and big data technologies like Hadoop and Spark, virtualization offers high flexibility in data integration and analysis. This technology is an ideal choice for companies looking to improve data management efficiency in the digital era. If you want to optimize data management with virtualization technology, Dave is here to help! Try our service and experience seamless data access and integration on a single platform.
Optimizing Analytics Processes with Data Virtualization
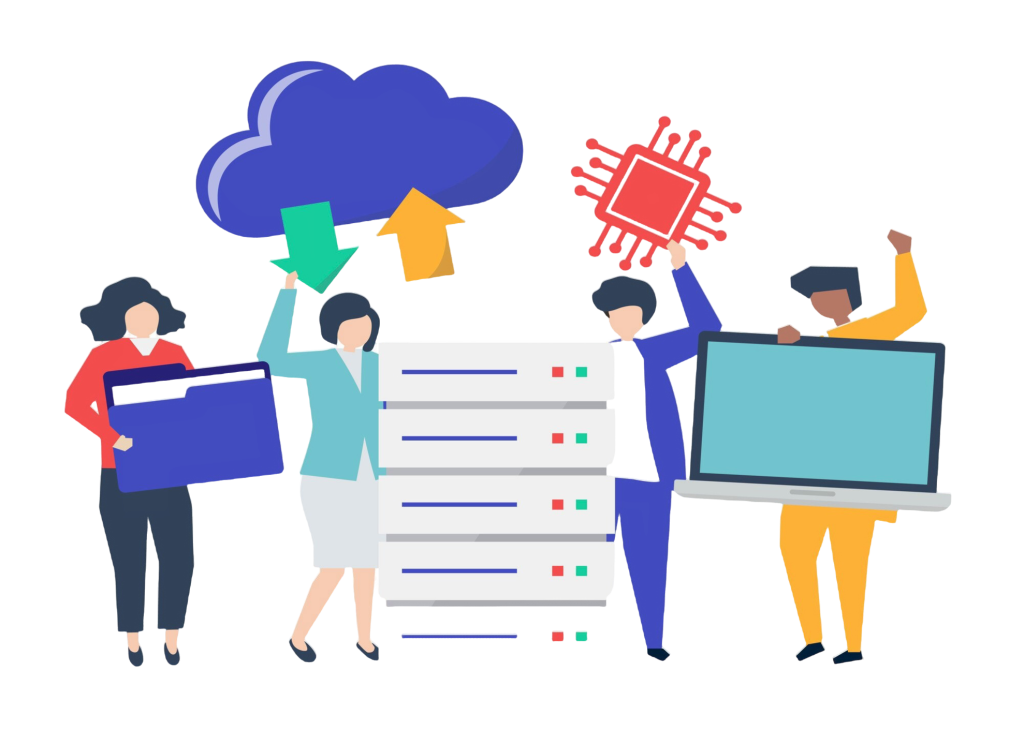
Introduction In the ever-evolving business landscape, data management has become a crucial factor in supporting better decision-making. Data virtualization is one of the technologies that helps accelerate and simplify analytics processes by integrating data from multiple sources. This article will discuss how data virtualization facilitates data integration, its role in Business Intelligence (BI), and the advantages and challenges faced in its implementation. What Is Data Virtualization? Data virtualization is a technology that enables data integration from multiple sources without the need to physically consolidate the data into a single repository. This means that data stays in its original source, and systems can still access and process it for analytics and reporting. By utilizing data virtualization, businesses access data from various applications simultaneously, even when different databases store those data sets. This technology employs metadata layers and semantic layers to present real-time data, reducing reliance on traditional ETL (Extract, Transform, Load) processes, which are often time-consuming. With this architecture, data virtualization also supports machine learning (ML) and advanced analytics. Read Also : Data Virtualization in Business Intelligence and Analytics Case Studies and Implementation in Various Industries Data virtualization has been adopted across various industries such as finance, retail, and healthcare to enhance analytics and expedite decision-making. The Role of Data Virtualization in Business Intelligence (BI) and Analytics Data virtualization plays a crucial role in self-service BI, enabling business users to access and analyze data without waiting for IT teams or data administrators to process it. Additionally, data virtualization facilitates real-time decision-making, as the data accessed is always updated directly from its source. Furthermore, data virtualization enables integration with data lakes, allowing organizations to manage dispersed data sources without losing analytical flexibility. Challenges in Processing Data from Multiple Applications Most organizations rely on various applications for their operational needs, such as sales, production, product management, and inventory systems. However, problems arise when data from these applications must be consolidated for analytical reporting. Since each application maintains its own database, merging this data for reporting purposes becomes increasingly complex. Traditionally, this process requires downloading data from multiple databases and combining it in a centralized location, often within a data warehouse. However, this approach can be time-consuming and burdensome, especially when dealing with large datasets. Consequently, the time required to generate accurate and timely reports increases, impacting business efficiency. Advantages of Using Data Virtualization Unlike traditional approaches that require physical data consolidation, data virtualization allows data to remain in its original source. Applications such as sales, inventory, and product management maintain their respective databases. However, with data virtualization, data processing and report generation can be performed directly without the need to download and transfer data to a centralized database. Key advantages of data virtualization include: These benefits are particularly valuable as data volumes continue to grow, ensuring that businesses can process and analyze data more quickly without overloading their systems. Conclusion Data virtualization is an effective solution for overcoming data integration challenges and accelerating analytics processes. By leveraging this technology, businesses can consolidate and process data from multiple applications in real-time without requiring physical data movement. By gaining a deeper understanding of data virtualization, companies can optimize data-driven decision-making and enhance their competitive edge in the digital era. If you would like to learn more about how this technology can benefit your business, contact us for a free consultation.
Practical Steps to Implement Data Virtualization and Optimization Tips
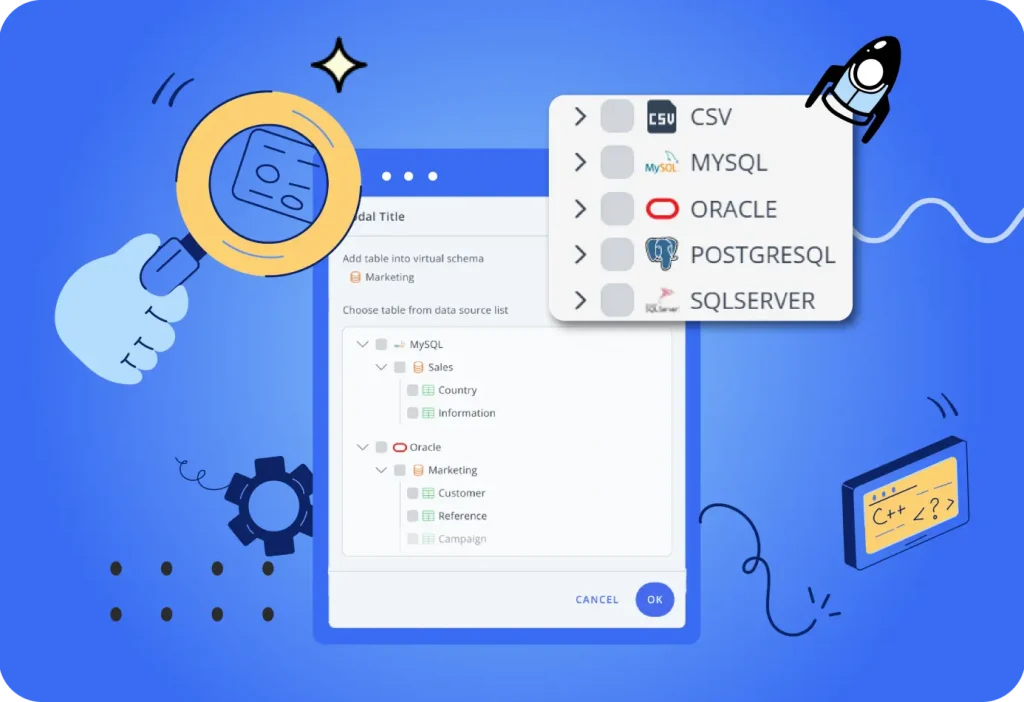
After understanding the differences between data virtualization and ETL and their benefits in managing data efficiently, the next question is: how do you get started? This article discusses the practical steps to implement data virtualization in your organization and provides optimization tips to maximize results. 1. Analyze Data Requirements and Existing Architecture Before starting, analyze business needs and the existing IT architecture. Additionally, consider potential future requirements to ensure scalability. Review: With this mapping, you can identify critical areas that need early integration. 2. Choose the Right Data Virtualization Platform and Technology Select a platform that fits your organization’s needs. Consider: If using a cloud-based service, make sure you understand the cost structure to avoid unexpected expenses. Read Also : What is Data Virtualization A Practical Guide 3. Design the Virtualization Model Once you’ve chosen a platform, build the virtualization model with the following steps. Next, ensure your team understands the model’s structure and purpose. This stage allows data analysis without physically moving the data. 4. Conduct Testing and Validation Before full implementation, conduct a proof of concept (PoC): This testing helps identify potential issues early on. 5. Optimize Performance and Resource Usage 6. Integrate with Analytics and BI Tools Connect the data virtualization layer with your analytics and BI tools, such as Tableau or Power BI. Ensure compatibility with internal applications that need real-time access. 7. Evaluate and Continuously Improve Perform regular evaluations by monitoring: Conclusion Data virtualization offers a practical solution for cross-application data integration while optimizing resource use. By consistently applying these steps, your organization can maximize data potential without the need to move or duplicate physical data excessively. If you would like to learn more about how this technology can benefit your business, contact us for a free consultation.
How Data Virtualization Helps Businesses Manage Data Faster and More Cost-Effectively
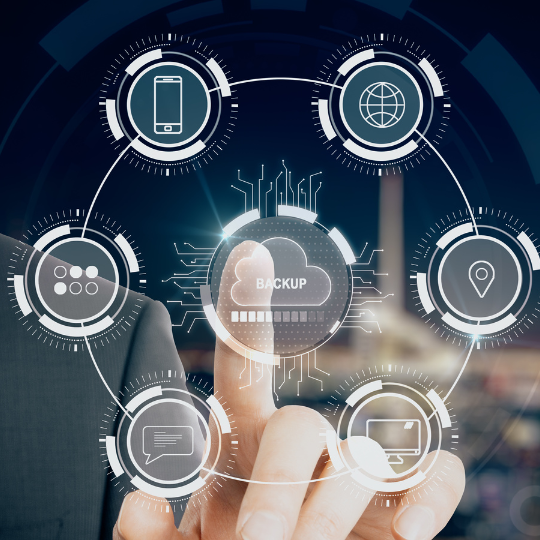
In today’s digital era, data stands as one of the most critical assets for any business. Yet, the increasing number of applications and data sources poses growing challenges in terms of data management, processing, and storage. Data virtualization emerges as a solution, enabling companies to integrate data efficiently without building costly infrastructure. This article explores how data virtualization can reduce duplication, cut down on storage and network demands, and streamline the consolidation of data across multiple applications. The Challenges of Traditional Data Management Let us consider four internal systems in a company: Finance, Sales, Marketing, and HR. Each system has its own database, resulting in four distinct databases. If we do not utilize data virtualization and instead build a data warehouse or employ other traditional methods, the aggregated data can grow extremely large, necessitating a robust network. The greater the demand for network speed and bandwidth, the higher the associated costs. In addition, the need for storage also increases. Why? Because a significant amount of data duplication occurs. For instance, data from Finance is copied into a Centralized Database, and the same happens with data from Sales, and so on. Further, each system requires archiving and backups, causing data to multiply: stored across each system, the Centralized Database, archives, and backups. Consequently, the need for storage capacity rises, and so do the costs. How Data Virtualization Reduces Storage and Network Costs Moreover, the computational (CPU) load on the Centralized Database can become excessively high. Data from Finance, Sales, Marketing, and HR, which may have already been processed at their respective sources, has to be reprocessed by the Centralized Database. This means more compute power is required to manage and analyze large-scale data. Meanwhile, with data virtualization, we only consolidate at “Dave”—the data virtualization engine. This setup automatically reduces network requirements, lowers storage needs, and eases computational burdens. Costs associated with traditional extract, transform, load (ETL) processes can thus be significantly curtailed. Imagine, as well, that these systems (Finance, Sales, Marketing, and HR) operate in the cloud. Without “Dave”, you would need to download each dataset individually, which is highly time-consuming. Conversely, if all systems—including “Dave”—are also in the cloud, they can “talk” to each other much faster since they share the same infrastructure, eliminating the need for an expensive physical network. Platforms like Azure, AWS, Google Cloud Platform (GCP), BigQuery, or Snowflake offer interconnected cloud services. By placing “Dave” in the cloud, you can leverage these services and avoid the overhead of constructing your own costly network. It is important to note that large-scale infrastructure is not only expensive to acquire, but also demanding in terms of maintenance. You need specialized teams to keep systems running, ensure security (to prevent cyberattacks), and maintain uptime. All these responsibilities can cause total costs to skyrocket if you rely on traditional approaches. You would need a robust network, large storage capacity, and significant computational power. By contrast, data virtualization lets you utilize existing resources more efficiently, reducing both the time and expense required for data management. Why Businesses Should Adopt Data Virtualization By implementing data virtualization, companies can accelerate data processing, decrease infrastructure costs, and optimize current resources. If you would like to learn more about how this solution can be applied to your business, contact us for a free demo or schedule a no-cost consultation. Together, let’s achieve the kind of data efficiency that drives growth and innovation in your organization!