Introduction
In the ever-evolving business landscape, data management has become a crucial factor in supporting better decision-making. Data virtualization is one of the technologies that helps accelerate and simplify analytics processes by integrating data from multiple sources. This article will discuss how data virtualization facilitates data integration, its role in Business Intelligence (BI), and the advantages and challenges faced in its implementation.
What Is Data Virtualization?
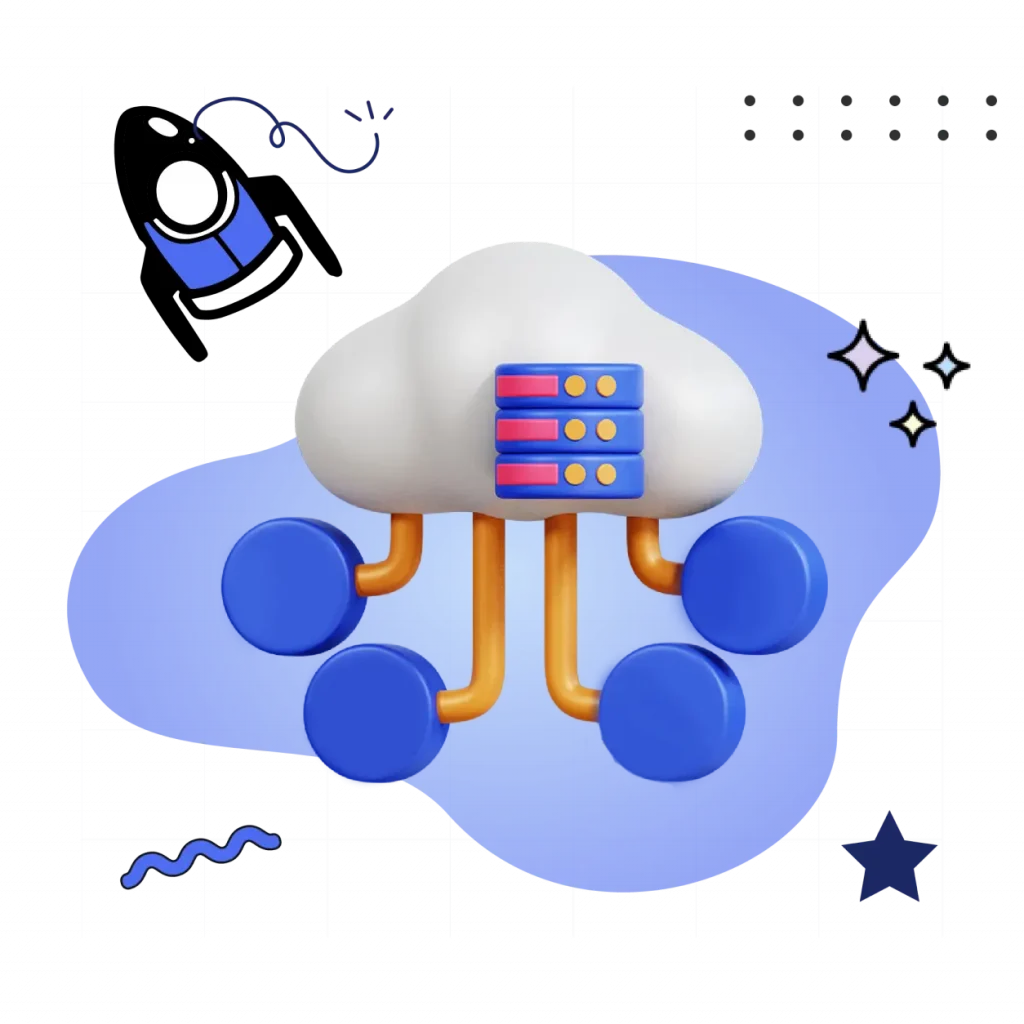
Data virtualization is a technology that enables data integration from multiple sources without the need to physically consolidate the data into a single repository. This means that data stays in its original source, and systems can still access and process it for analytics and reporting. By utilizing data virtualization, businesses access data from various applications simultaneously, even when different databases store those data sets.
This technology employs metadata layers and semantic layers to present real-time data, reducing reliance on traditional ETL (Extract, Transform, Load) processes, which are often time-consuming. With this architecture, data virtualization also supports machine learning (ML) and advanced analytics.
Read Also : Data Virtualization in Business Intelligence and Analytics
Case Studies and Implementation in Various Industries
Data virtualization has been adopted across various industries such as finance, retail, and healthcare to enhance analytics and expedite decision-making.
- Finance: Banks use data virtualization to unify data from different transaction systems and analyze it in real-time.
- Retail: Retail companies integrate sales and inventory data from multiple branches without physically consolidating the data.
- Healthcare: Hospitals and healthcare providers use data virtualization to access patient medical records from various sources.
The Role of Data Virtualization in Business Intelligence (BI) and Analytics
Data virtualization plays a crucial role in self-service BI, enabling business users to access and analyze data without waiting for IT teams or data administrators to process it. Additionally, data virtualization facilitates real-time decision-making, as the data accessed is always updated directly from its source.
Furthermore, data virtualization enables integration with data lakes, allowing organizations to manage dispersed data sources without losing analytical flexibility.
Challenges in Processing Data from Multiple Applications
Most organizations rely on various applications for their operational needs, such as sales, production, product management, and inventory systems. However, problems arise when data from these applications must be consolidated for analytical reporting. Since each application maintains its own database, merging this data for reporting purposes becomes increasingly complex.
Traditionally, this process requires downloading data from multiple databases and combining it in a centralized location, often within a data warehouse. However, this approach can be time-consuming and burdensome, especially when dealing with large datasets. Consequently, the time required to generate accurate and timely reports increases, impacting business efficiency.
Advantages of Using Data Virtualization
Unlike traditional approaches that require physical data consolidation, data virtualization allows data to remain in its original source. Applications such as sales, inventory, and product management maintain their respective databases. However, with data virtualization, data processing and report generation can be performed directly without the need to download and transfer data to a centralized database.
Key advantages of data virtualization include:
- Improved Efficiency: Eliminates the need for time-consuming ETL processes.
- Faster Report Generation: Reduces the time needed to compile and analyze data.
- Less System Overload: Reduces the burden on infrastructure by avoiding redundant data storage.
- Scalability: Enables organizations to manage and analyze larger datasets more effectively.
These benefits are particularly valuable as data volumes continue to grow, ensuring that businesses can process and analyze data more quickly without overloading their systems.
Conclusion
Data virtualization is an effective solution for overcoming data integration challenges and accelerating analytics processes. By leveraging this technology, businesses can consolidate and process data from multiple applications in real-time without requiring physical data movement.
By gaining a deeper understanding of data virtualization, companies can optimize data-driven decision-making and enhance their competitive edge in the digital era.
If you would like to learn more about how this technology can benefit your business, contact us for a free consultation.