In today’s digital era, data stands as one of the most critical assets for any business. Yet, the increasing number of applications and data sources poses growing challenges in terms of data management, processing, and storage. Data virtualization emerges as a solution, enabling companies to integrate data efficiently without building costly infrastructure. This article explores how data virtualization can reduce duplication, cut down on storage and network demands, and streamline the consolidation of data across multiple applications.
The Challenges of Traditional Data Management
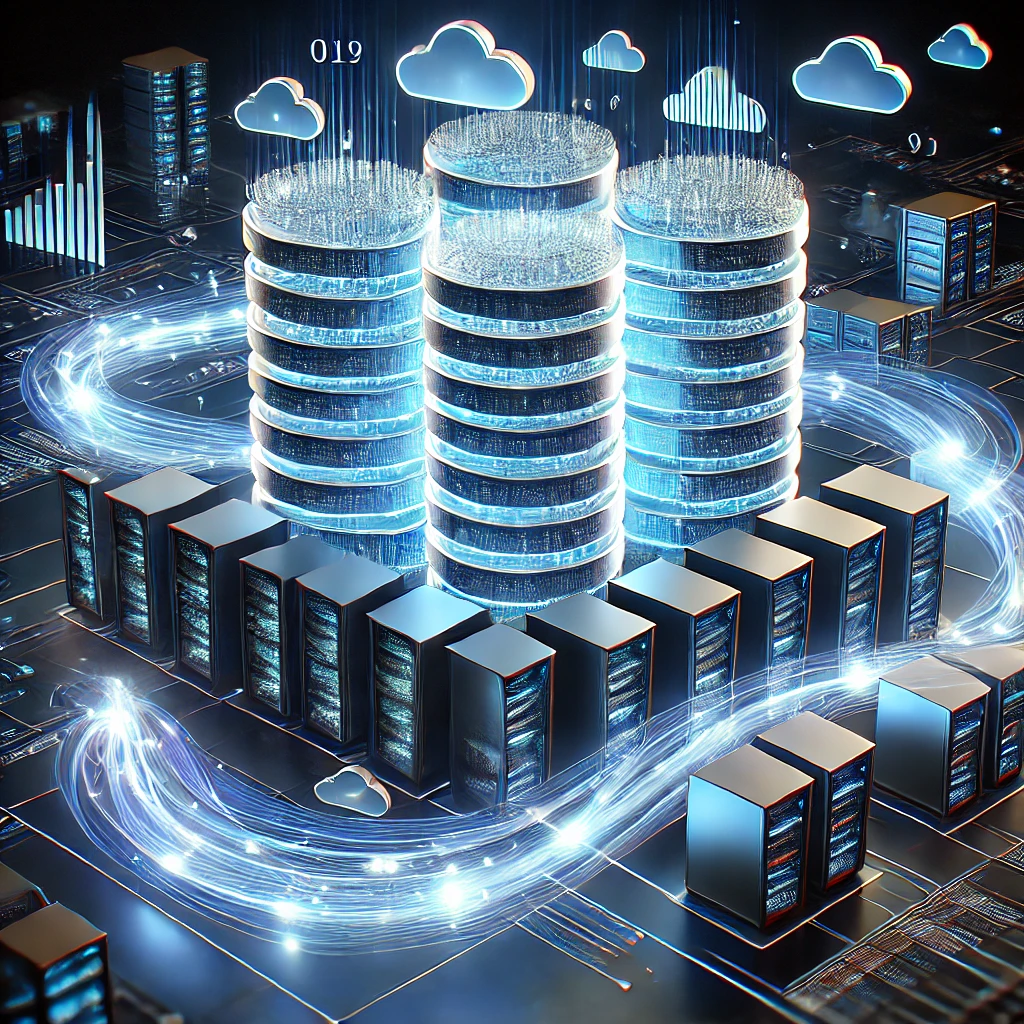
Let us consider four internal systems in a company: Finance, Sales, Marketing, and HR. Each system has its own database, resulting in four distinct databases. If we do not utilize data virtualization and instead build a data warehouse or employ other traditional methods, the aggregated data can grow extremely large, necessitating a robust network. The greater the demand for network speed and bandwidth, the higher the associated costs.
In addition, the need for storage also increases. Why? Because a significant amount of data duplication occurs. For instance, data from Finance is copied into a Centralized Database, and the same happens with data from Sales, and so on. Further, each system requires archiving and backups, causing data to multiply: stored across each system, the Centralized Database, archives, and backups. Consequently, the need for storage capacity rises, and so do the costs.
How Data Virtualization Reduces Storage and Network Costs
Moreover, the computational (CPU) load on the Centralized Database can become excessively high. Data from Finance, Sales, Marketing, and HR, which may have already been processed at their respective sources, has to be reprocessed by the Centralized Database. This means more compute power is required to manage and analyze large-scale data. Meanwhile, with data virtualization, we only consolidate at “Dave”—the data virtualization engine. This setup automatically reduces network requirements, lowers storage needs, and eases computational burdens. Costs associated with traditional extract, transform, load (ETL) processes can thus be significantly curtailed.
Imagine, as well, that these systems (Finance, Sales, Marketing, and HR) operate in the cloud. Without “Dave”, you would need to download each dataset individually, which is highly time-consuming. Conversely, if all systems—including “Dave”—are also in the cloud, they can “talk” to each other much faster since they share the same infrastructure, eliminating the need for an expensive physical network. Platforms like Azure, AWS, Google Cloud Platform (GCP), BigQuery, or Snowflake offer interconnected cloud services. By placing “Dave” in the cloud, you can leverage these services and avoid the overhead of constructing your own costly network.
It is important to note that large-scale infrastructure is not only expensive to acquire, but also demanding in terms of maintenance. You need specialized teams to keep systems running, ensure security (to prevent cyberattacks), and maintain uptime. All these responsibilities can cause total costs to skyrocket if you rely on traditional approaches. You would need a robust network, large storage capacity, and significant computational power. By contrast, data virtualization lets you utilize existing resources more efficiently, reducing both the time and expense required for data management.
Why Businesses Should Adopt Data Virtualization
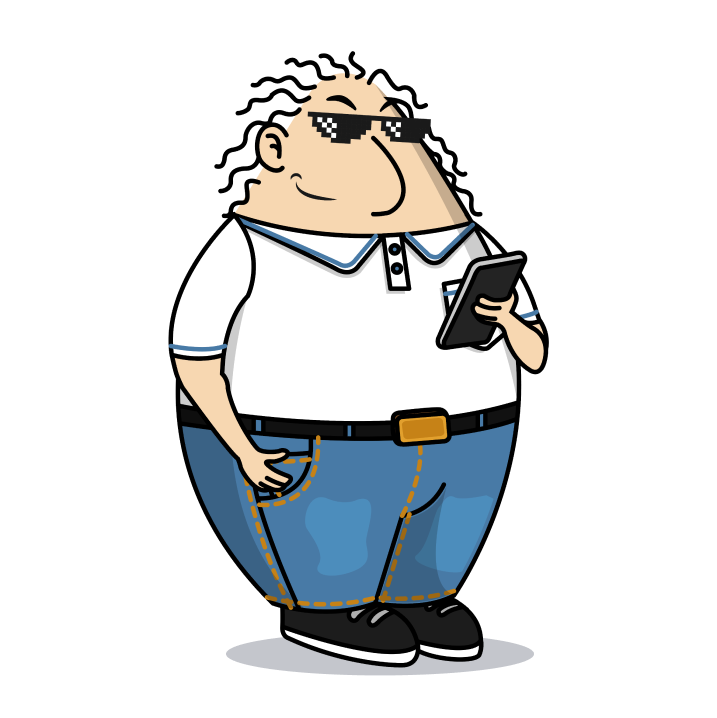
By implementing data virtualization, companies can accelerate data processing, decrease infrastructure costs, and optimize current resources. If you would like to learn more about how this solution can be applied to your business, contact us for a free demo or schedule a no-cost consultation. Together, let’s achieve the kind of data efficiency that drives growth and innovation in your organization!